What is Explainable AI?
August 7, 2022
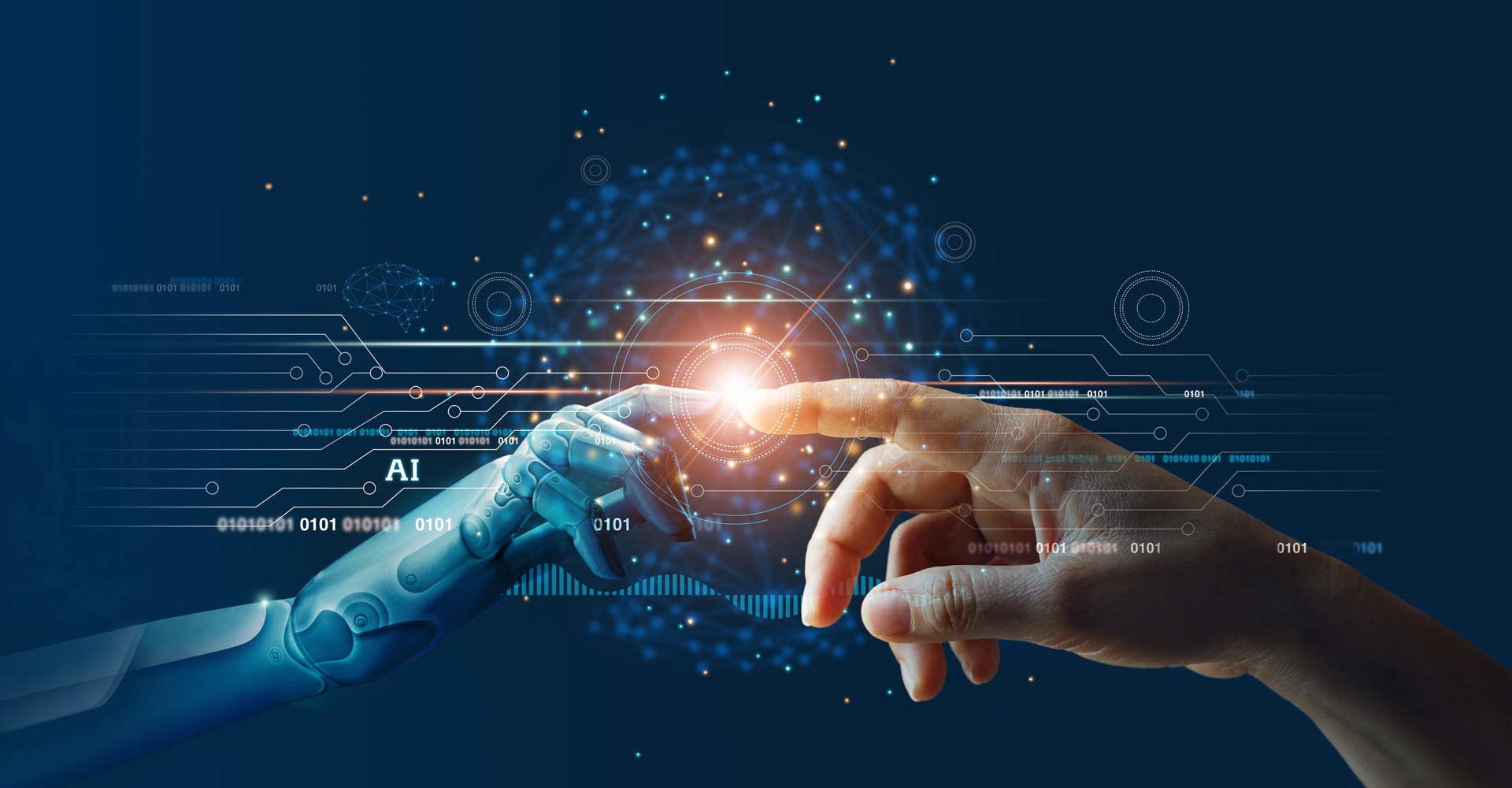
In today’s digital world, Artificial Intelligence (AI) plays a major role in how businesses operate. But understanding the decisions made by machines can be difficult—even for experts. This is where Explainable AI (XAI) comes in. XAI is designed to make complex machine decisions more understandable and transparent to all stakeholders in the process.
Let’s take a closer look at XAI and its importance for businesses.
What Is Explainable AI?
Explainable AI (XAI) is a revolutionary concept that helps bridge the gap between artificial intelligence and human understanding. By breaking down complex decision processes into simpler, more understandable steps, XAI gives non-technical audiences insight into how automated or semi-automated decisions are made.
Not only does it provide explanations for why certain models or parameters were chosen, but also provides invaluable insights that could otherwise be overlooked. With XAI, we are able to take mechanical learning one step closer to understanding what humans intuitively do naturally.
Challenges to the Explainability of Intelligent Systems
Deep learning algorithms and their use in creating models for AI systems have added an additional layer of complexity to XAI. Due to their intricate structures, combined with the potentially biased data they may have been trained on, it can be difficult to explain why a certain AI-based decision was made without sacrificing accuracy.
Thus, stakeholders are left unable to gain insight into what went into a machine’s decision-making process or whether biases played a role in the results. To truly reap the benefits of XAI, these challenges must be addressed and overcome.
In addition, there are other issues that can complicate the explainability of AI systems. Algorithms must be designed in a way that allows them to provide transparent explanations without sacrificing accuracy or speed. Any data used to build the system must be collected responsibly and ethically to ensure fairness and accuracy.
Why Is XAI Important for Business?
As businesses become increasingly reliant on automated or semi-automated decision-making systems, it becomes increasingly important that those decisions are both accurate and explainable to stakeholders across the organization.
Without an explanation of why a system made a particular decision, companies may find themselves unable to defend their choices if they are called into question later on down the line. Having an explainable system makes it easier for organizations to audit their own processes and identify potential areas of improvement or bias within their models—which could lead to better decision-making overall.
AI Explainability Techniques
The techniques for explaining AI-based decisions vary greatly depending on the algorithm and system in question. Generally speaking; however, there are several common approaches to explainability.
Rule-based explanations are one of the simplest and most effective techniques used to explain AI systems. This technique uses a set of rules that describe how certain data points are related to each other or to certain outcomes. For example, a rule-based explanation might reveal that people with higher incomes tend to buy more expensive cars than those with lower incomes.
These rules can help researchers identify patterns in data and provide simple, clear results that stakeholders can understand. They also allow for easier debugging of machine learning models, since errors in the model can be easily traced back to a specific rule or set of rules.
Feature attribution techniques are slightly more complex than rule-based explanations but still relatively easy to use and understand. These techniques involve assigning weights or values to certain features or parameters in order to better understand how they affect the system’s output.
For example, if we wanted to know which feature had the biggest impact on a model’s predictions, we could assign weights or values to each feature and then compare them against each other in order to determine which was most influential.
Feature visualization techniques are even more advanced than feature attribution methods but still offer valuable insights into how an AI system works. These techniques involve creating graphical representations of model parameters and their associated weights so that stakeholders can gain a better understanding of how certain decisions were made by the system.
Feature visualizations can also help researchers detect any potential bias in the model by highlighting relationships between different features or parameters that might not have been obvious before.
How Can Organizations Use XAI?
Organizations can use XAI in a variety of ways depending on their needs and goals. For example, some organizations may use XAI as part of their data governance strategy in order to ensure that all data is accurately represented and explained within the system.
Others may use it as part of auditing procedures in order to gain insight into how different models are being used across different departments or teams within their organization. And still others may use it simply as a way of increasing transparency around automated decision-making within their organization—particularly when those decisions have an impact on customers or other external stakeholders.
How Does XAI Benefit Businesses?
XAI has the potential to revolutionize how businesses operate. Here are some of the ways XAI can benefit businesses:
One of the biggest advantages of using XAI is improved transparency in decision-making processes. By understanding exactly how an AI system reached its conclusions, businesses can quickly identify potential errors or biases and make adjustments accordingly. This helps to ensure that decisions are made based on accurate data and evidence rather than guesswork or intuition alone.
With improved transparency comes improved decision-making capabilities. Companies can use the insights gained from understanding an AI system’s decision-making process to make more informed decisions with greater confidence. This leads to better results in terms of efficiency and effectiveness as well as customer satisfaction levels.
Automation enabled by XAI significantly reduces costs associated with manual labor, freeing up resources for other initiatives such as product development or marketing campaigns. This helps businesses remain competitive in their respective industries while still achieving their goals without breaking the bank on labor costs.
Using XAI to automate decisions can lead to increased customer satisfaction. By providing customers with explanations for automated decisions, businesses can ensure that customers feel more connected to their products and services, leading to better customer loyalty in the long run.
How To Build Trust In XAI
In order for XAI to be trusted, it is essential for organizations to ensure social transparency when deploying the technology. Social transparency requires that stakeholders such as customers, employees, and other stakeholders understand exactly how an AI system works and makes decisions. This includes not only providing explanations of the decisions made by an AI system but also actively engaging with stakeholders to answer any questions they may have.
Explainability Strategies
Explainability strategies refer to the methods used to explain how an AI decision was made. Explainability approaches can make complex models more understandable for non-technical audiences, which can help build trust within a company or organization.
For example, a company that deploys XAI (eXplainable Artificial Intelligence) could provide regular reports on model performance and accuracy, as well as engage stakeholders in feedback loops throughout the life of the project. This will ensure that everyone involved understands how XAI algorithms work and is able to verify that the system is working correctly.
Additionally, user-friendly visualizations can be used to make complex models more approachable for a general audience. These visualizations can also be used to highlight any potential risks associated with using XAI technology.
Audit Trails and Performance Insights
Another way to increase trust in XAI is through audit trails and performance insights. By having an embedded audit trail in each decision-making model, it allows developers and stakeholders to monitor its performance over time without having to manually track every detail of each decision-making process.
Furthermore, by providing performance insights into how each model performs against different scenarios or data sets gives stakeholders more visibility into its overall effectiveness which increases trust in its output.
Ethical Considerations of Explainable AI
XAI introduces a new set of ethical considerations that need to be taken into account when implementing AI-driven decision-making processes. As with any technology, there is the potential for it to be misused or abused, and XAI is no exception.
Organizations should make sure they are considering issues such as privacy, fairness, accountability, and transparency when designing and building their XAI models. Additionally, organizations should ensure they are providing clear explanations for automated decisions in order to ensure customers feel well-informed and empowered to make the best decisions for themselves.
By taking these steps, organizations can use Explainable Artificial Intelligence (XAI) to their advantage while still being mindful of its ethical implications. By leveraging XAI, businesses can make better decisions and provide a higher level of customer service while still ensuring that their models are compliant with ethical standards.
Conclusion
Explainable AI (XAI) is an incredibly important tool for businesses who want to increase transparency around automated decisions and make sure that those decisions accurately reflect real-world conditions and outcomes.
By providing insight into how different models are being used across different departments or teams within an organization, XAI can be instrumental in helping companies audit their own processes, identify potential areas of improvement or bias within their models, and ultimately make better decisions overall—all while increasing transparency around automated decision making along the way.
ABOUT MORANT MCLEOD
Our management consulting processes are a highly effective way for businesses to improve their organizational performance and achieve their goals. We offer expertise and specialized tools that can help organizations navigate the challenges involved in major changes.